The Wolfsberg principles for the responsible use of AI and ML in financial crime compliance: a practical guidance
The Wolfsberg Group, an association of thirteen global banks, delivers highly respected expertise and guidance in the fields of financial crime, including anti-money laundering (AML) and counter terrorist financing (CTF). It develops frameworks and guidelines that help financial institutions manage financial crime risks, and has recently published a set of principles for using artificial intelligence (AI) and machine learning (ML) in financial crime compliance.
These five principles support financial institutions in the responsible implementation of AI in financial crime compliance applications. Read on to discover what you can do in practice.
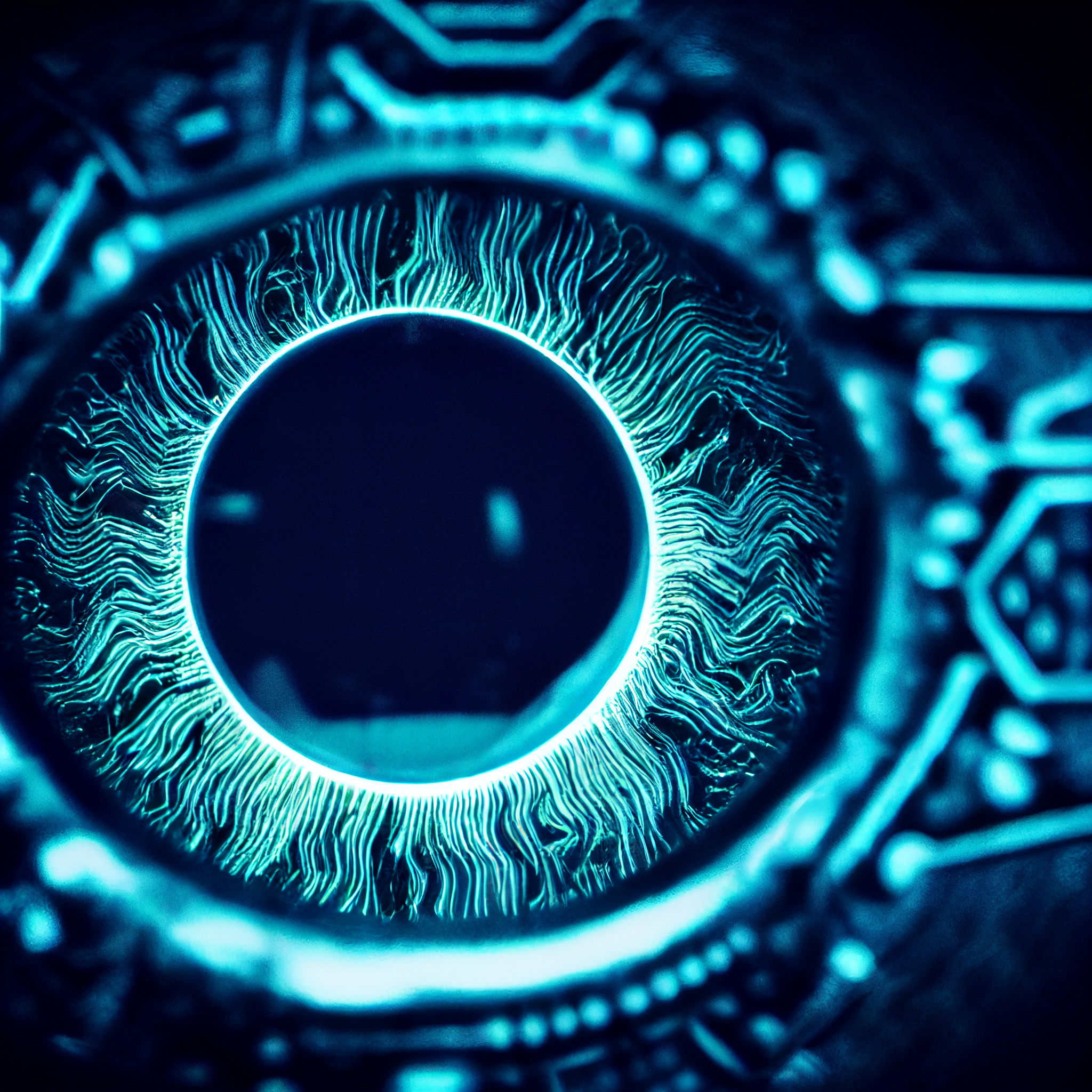
The Wolfsberg Group, an association of thirteen global banks, delivers highly respected expertise and guidance in the fields of financial crime, including anti-money laundering (AML) and counter terrorist financing (CTF). It develops frameworks and guidelines that help financial institutions manage financial crime risks, and has recently published a set of principles for using artificial intelligence (AI) and machine learning (ML) in financial crime compliance.
These five principles support financial institutions in the responsible implementation of AI in financial crime compliance applications. Read on to discover what you can do in practice.
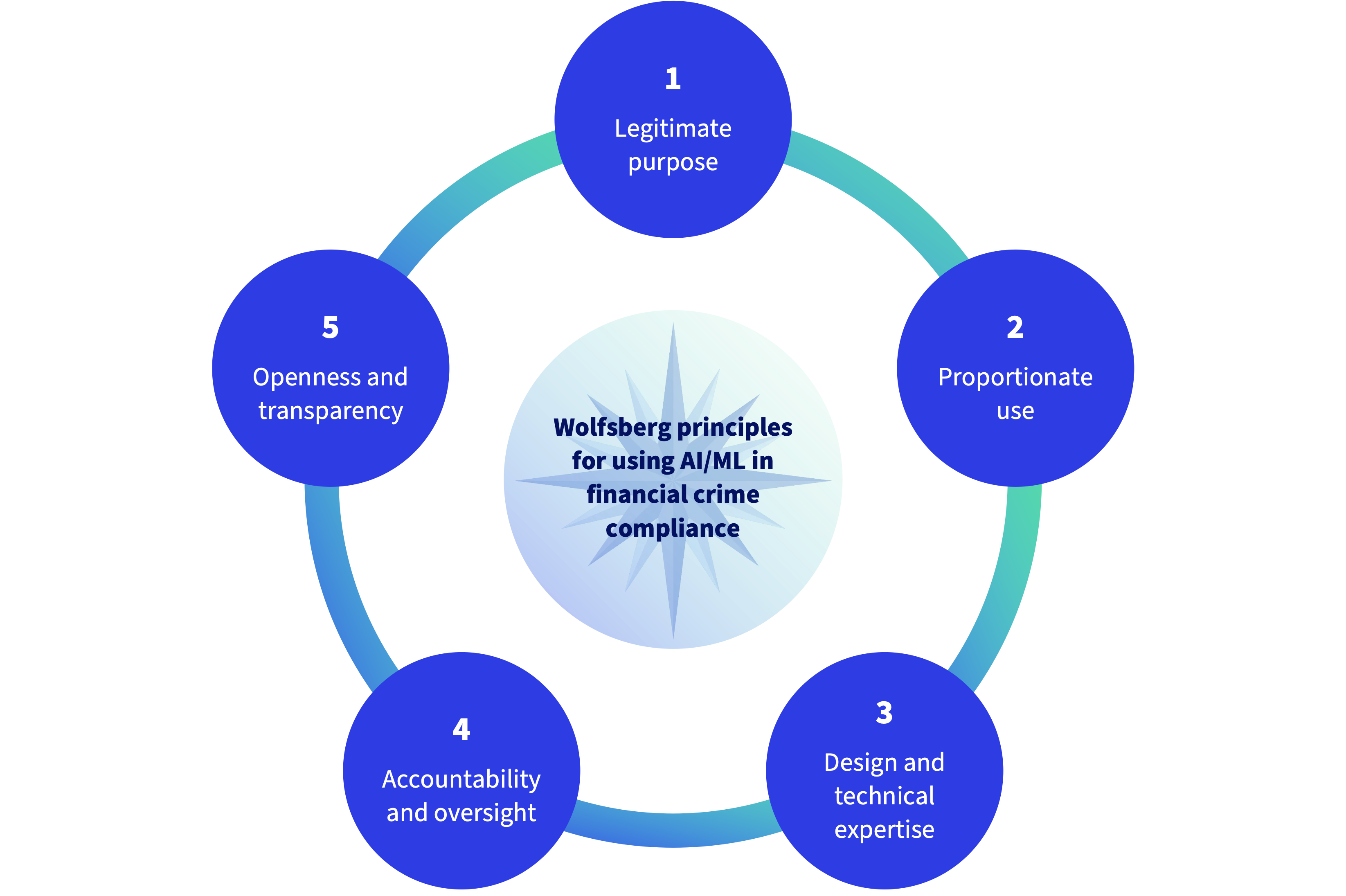
Principle 1: Legitimate purpose
The battle against financial crime doesn’t just serve the financial system, it also benefits society as a whole. Preventing money laundering makes it difficult for criminals to use illegal earnings in the financial system, which means it’s a lot harder for them to run their ‘businesses’.
The use of AI and ML is already making AML investigations more efficient and more effective. And the regulatory community has certainly noticed. The National Bank of the Netherlands has developed an AI-based transaction monitoring tool that challenges the tools used by financial institutions. In its ‘Vision on supervision 2021–2024’, the regulator declared the use of data crucial for efficient and effective supervisory monitoring. And in a keynote speech delivered in 2021, the Monetary Authority of Singapore predicted that the use of data analysis techniques will be a mainstay in the future AML/CTF landscape. It also advises financial institutions to equip their AML/CTF professionals with the skills needed to make full use of these promising tools.
These techniques, however, must be used responsibly. Financial institutions need to create a balanced mix of AML performance, data protection, data security and the responsible use of AI.
When AI/ML models are implemented, several activities are typically performed to ensure they do not unintentionally cause discrimination, operational risks or the unfair use of data:
- Model validation – Evaluating the model itself and the context and processes in which it is implemented. This reveals how well the model performs with previously unseen data (robustness), as well as how sensitive it is to changes in the data and whether it can distinguish the ‘good guys’ from the ‘bad guys’ effectively.
- Data stability and quality monitoring – Ensuring stable dataflows in both model training and periodic runs of the model, usually including both input and output data monitoring.
- Fair AI assessment – Testing of the AI’s sensitivity towards discriminative factors such as gender or ethnicity. Although some discriminative factors might be considered important in the investigation (e.g. nationality vs destination of funds), others are irrelevant and should not be part of the screening (e.g. sexual orientation).
- The models are typically added to a model inventory, where model owners and review schedules are agreed upon. This usually includes risk categorisation of the models, which results in a risk-based approach for maintenance and monitoring of the model, in line with the risk-management framework that is used in the organisation.
Principle 2: Proportionate use
AI/ML models are designed to help; they shouldn’t lead to risks or consequences that outweigh the added value of using them. For example, if a model is used to assess the likelihood of a client being involved in payment fraud and this means the client is automatically reported to the police and/or the client relationship is terminated, the model’s prediction had better be right.
Several methods are used to ensure proportionate use of AI/ML models:
-
Keeping a human involved in decisions
Decisions that can have far-reaching consequences for the client should not be automated. AI/ML models always have a margin of error, that’s why a human being should always make the final decision (at least when the error margin is above a certain level). -
Regular reviews of input parameter configuration
The data that are processed by the AI/ML models, like lists of risky countries or professions, or financial parameters, are (obviously) very important. Regular reviews and updates of the parameters defining these data are advised. Significant changes might indicate a need for AI/ML models to be retrained. -
Supervised learning
AI/ML models can use historical financial crime cases to identify crimes today. This works very well for crimes that occur frequently, but isn’t optimal for types of financial crime that are new or have not been frequently observed in the past. In these cases, unsupervised learning or even rule-based solutions can be good alternatives. -
Data protection and data security protocols
Safe protocols for transferring, storing, and processing data are considered hygiene factors in financial crime fighting solutions. They can mitigate the risk of data breaches and their consequences. Moreover, data minimisation and pseudonymisation further mitigate these risks.
Principle 3: Design and technical expertise
Proper understanding of AI/ML models that are in use and how they are being implemented is critical for ensuring responsible usage. Multiple people are involved in the design and implementation of AI/ML models. They’re responsible for different tasks and require specific information, for example:
- Compliance investigators in the AML team need to know why a particular trigger was created and how certain the model was about the assessment.
- Compliance or risk executives benefit from knowing the strengths and weaknesses of working with AI/ML in general, as well as the results of regular validation exercises.
- Model validation teams should have an in-depth view of the algorithms and assumptions used in a model, and the context in which the model was implemented. If external vendors are used, clear agreements should be made regarding the roles and responsibilities in this area.
Principle 4: Accountability and oversight
It’s imperative that every financial institution knows exactly which AI/ML models are being used within the organisation. Typically, the model owner is responsible for monitoring the model’s performance, conducting periodic retraining and validation, analysing ethical and fair use of AI, and updating the model inventory. In larger organisations, these responsibilities might be assigned to different people.
It’s important to note that it’s not just the model owner who takes responsibility, but also the compliance and risk executives, who hold final accountability for the responsible and correct use of AI/ML models.
Principle 5: Openness and transparency
Openness and transparency in the use of AI is about creating the right balance. On one hand, regulators and risk executives should be well aware of where AI/ML is used and have an understanding of what it does. Moreover, customers should be informed that their data is being used for the purpose of financial crime fighting. This is usually done via privacy statements and is typically dependent on local legislations like the General Data Protection Regulation (GDPR) requirements in Europe.
On the other hand, too much openness could lead to unwanted consequences. For example, if a financial institution is too transparent about the features of AI/ML models used for the purpose of AML or fraud, customers with bad intentions could use that information to bypass or manipulate the monitoring system. One commonly used technique is to cut transactions into smaller pieces (smurfing) to avoid being flagged by traditional rule-based AML tools with thresholds on the transaction amount.
The role of the software vendor
As a financial institution, you should not hesitate to ask your software vendor questions regarding the responsible, fair, secure, and qualitative use of AI or ML. Although financial crime fighting is all about differentiating the ‘good guys’ from the ‘bad guys’, it’s important to remain vigilant in monitoring the potential for unintentional discrimination. In some regions (e.g. Europe), ongoing legislative initiatives provide more concrete guidance about this. Moreover, model risk management frameworks should be applied to both internally developed models and models that are provided by external software vendors.
How Discai’s AML ensures the responsible use of AI/ML models
AI/ML models can take the battle against financial crime to a whole new level, but this should always be done in a responsible, secure, and qualitative manner. At Discai, with our roots in KBC Group, we follow all the requirements from regional and local regulators, as well as factoring in internal risk policies when developing our solutions. And we have measures in place to ensure this:
- Every generic product offered by Discai has been evaluated by KBC Group’s fair AI assessment tool, preparing it to comply with Europe’s regulatory framework on AI. We encourage our customers to perform their own assessment before local implementation, for which support can be provided.
- We provide regular retraining of all models, as well as technical and data stability monitoring.
- Although we have significantly reduced the number of false positives in the AML investigation process, we realise there will still be triggers activated that will not lead to a suspicious activity report (SAR). The Discai KYT AML solution still puts a human in the loop of the decision-making. The final decision on whether or not to report to the relevant financial intelligence unit (FIU) is a human decision, supported by AI.
- Every generic Discai solution has a dedicated model owner who acts as a single point of contact (SPOC) for the model owner on the customer side. This SPOC is available to act as a sparring partner to discuss potential model retraining, validation exercises and business performance monitoring.
- Every solution adheres to strict data protection and data security requirements. This includes data minimisation, data separation between different entities, data encryption and pseudonymisation, secure data transfer, storage and processing, and much more.
Want to use the Wolfsberg principles to take AML to the next level?
Let us show you how