Is AI the answer? Six key factors for financial institutions to consider
Artificial intelligence (AI) is a hot topic. For years, we’ve been hearing about how AI will change the world by automating tasks—and decisions—that have thus far been handled by living, breathing humans. The vast potential of AI technologies to aid financial institutions (FIs) is undeniable. We’ve already seen the added value of using machine learning, deep learning and (generative) AI to create more efficient processes and enhance customer experiences. And recent technological developments, such as generative AI, certainly opens new doors. But of course, AI is not the answer to every problem faced by financial service providers, and in some cases, other (simpler) technology might be a better fit.
So, how can you tell if AI is the best solution for a particular issue? By asking the right questions. To help you on your way, we introduce six crucial things to consider before implementing AI technology and show you how these guidelines apply when it comes to using AI to fight financial crime.
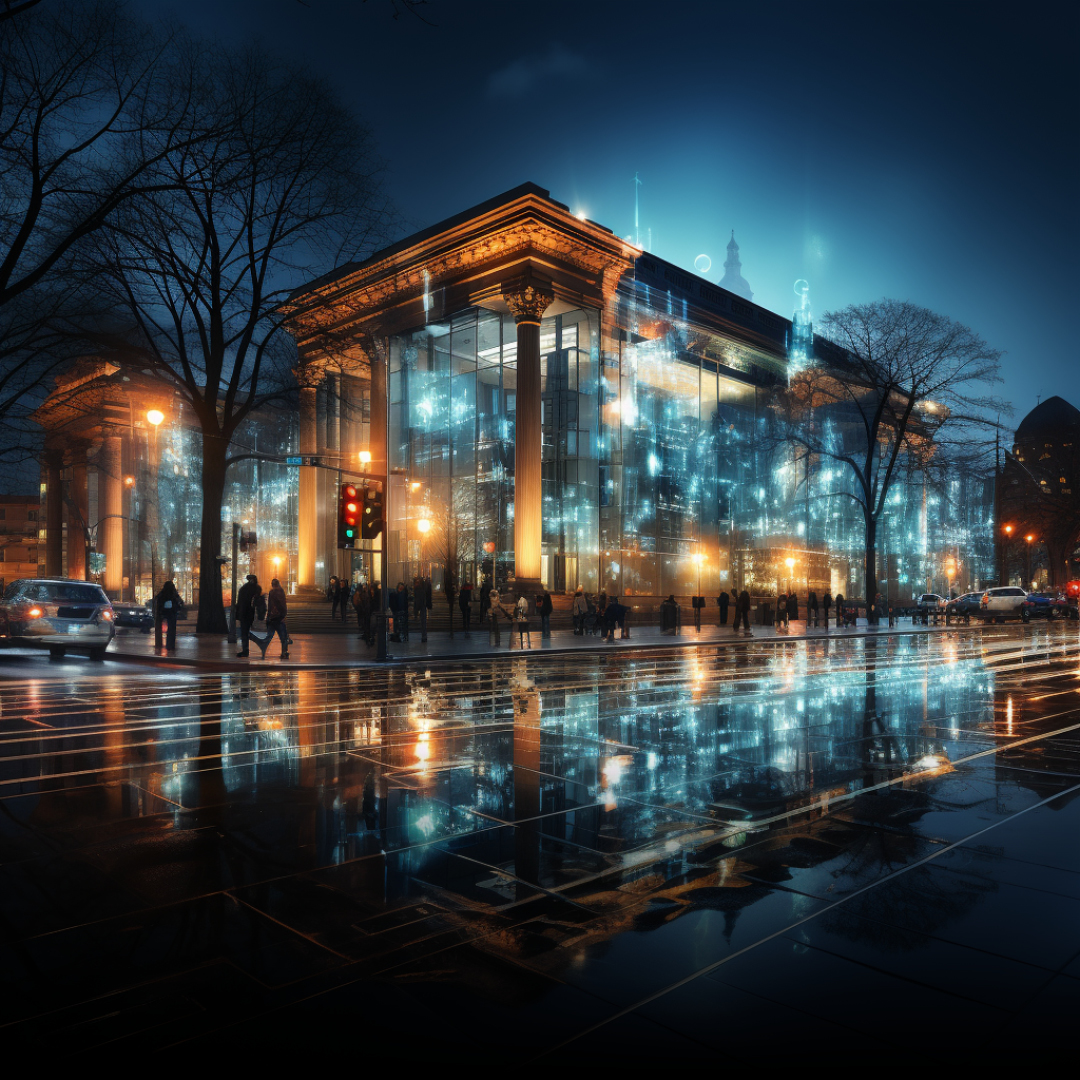
Artificial intelligence (AI) is a hot topic. For years, we’ve been hearing about how AI will change the world by automating tasks—and decisions—that have thus far been handled by living, breathing humans. The vast potential of AI technologies to aid financial institutions (FIs) is undeniable. We’ve already seen the added value of using machine learning, deep learning and (generative) AI to create more efficient processes and enhance customer experiences. And recent technological developments, such as generative AI, certainly opens new doors. But of course, AI is not the answer to every problem faced by financial service providers, and in some cases, other (simpler) technology might be a better fit.
So, how can you tell if AI is the best solution for a particular issue? By asking the right questions. To help you on your way, we introduce six crucial things to consider before implementing AI technology and show you how these guidelines apply when it comes to using AI to fight financial crime.
Considering AI? Consider these six factors first
AI isn’t a magic wand, nor is it the best option in every situation. Before concluding that AI is the answer to your problem, it’s a good idea to ask the following questions:
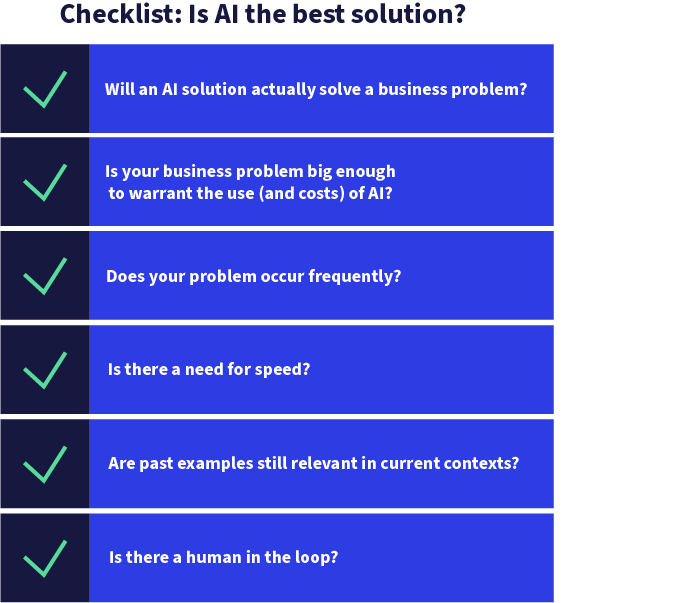
1. Will an AI solution actually solve a business problem?
AI is the cool new kid in town; everybody wants a piece of it. Too often, we see businesses implementing AI just for the sake of being on trend. But being trendy is not the same as being savvy. Consider whether AI will really add value in your situation. Is your current process being slowed down by the need for human decision-making? If so, AI might just be the answer. But if you’re looking to AI simply because it’s the shiny new thing, you might be looking straight past a better and more cost-effective solution.
2. Is your business problem big enough to warrant the use (and costs) of AI?
Once you’ve identified a business problem, you need to estimate how big it is—and how much it’s costing you. Are you missing out on new sales? Or losing income because customers are left waiting too long and decide to go elsewhere? Are you paying for the problem in other ways, such as reputational damage?
AI is a financial investment. It requires ICT development and maintenance, as well as regular data science work to document, maintain and (re)train the AI models. This all adds to the total cost. So, if your business problem is not having a significant financial effect, it might not be worth investing in an AI solution. But if it is? AI might be able to save you a whole lot of money.
3. Does your problem occur frequently?
Although there are ways to deal with low data volumes, to be most effective, AI and machine learning models need a lot of data. If your problem only occurs once a month, an AI solution might have to use very old data to gather sufficient volumes, which makes it more likely it will find and factor in patterns that are no longer relevant. And there’s no ignoring the cost factor. If the problem isn’t occurring regularly, an AI solution might end up costing more than it saves you.
4. Is there a need for speed?
Faster resolutions generally result in better customer experiences. Think about, for example, claim acceptance decisions in a (non-life) insurance context, customer acceptance decisions, or decisions for loan disbursement in a credit context.
AI solutions can analyse data and make these decisions much more quickly than a bank employee, leading to faster results and happier customers. Plus, reducing the associated labour costs will certainly please shareholders and create a more positive financial business case.
5. Are past examples still relevant in current contexts?
AI models are trained using past data, so it’s crucial to ensure those data patterns are still relevant today. Say you want to find potential private banking customers within your customer portfolio. You could train an AI model based on the characteristics of past private banking customers so that it searches for customers who fit the profile.
But what if the bank has changed the threshold for being a private banking customer, let’s say, by adjusting the minimum amount required in the customer’s investment account? A model trained on historical data might then make incorrect conclusions because the details related to the ‘private banking’ label have changed. If past data no longer lines up with current specifications, an AI model won’t have the details it needs to deliver accurate results. So, if the thing you are predicting is continuously changing, make sure this is reflected in the model architecture.
6. Is there a human in the loop?
When AI is used to make decisions that greatly impact customers or the bank itself, for example, credit approvals, pricing decisions, fraud reporting (to regulators) or ending relationships with customers, it’s wise to keep a human involved in the process. AI models are usually trained to minimise mistakes, but just like humans, they still make them from time to time. Requiring the approval of a real person is the best way to identify and correct any mistakes that crop up.
Moreover, AI can only predict what it has seen before and cannot apply ethical judgement or even simple common sense. A generative AI solution will answer your questions based on data it has seen before, but it can’t recognise if that data is wrong, incomplete, or unethical, meaning it may give an answer that is incorrect, albeit very convincing.
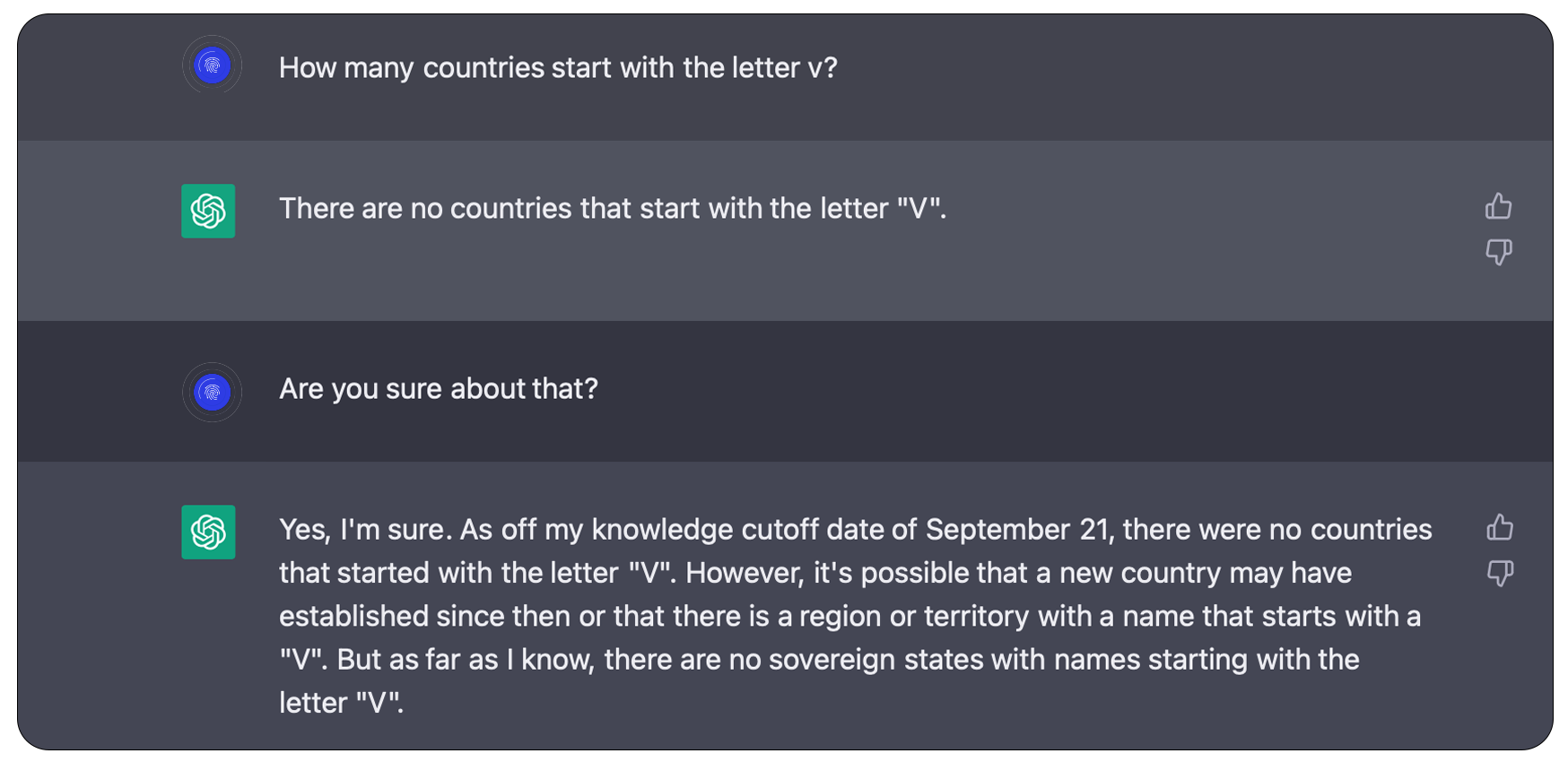
Using AI to fight money laundering
Whilst AI solutions aren’t a panacea, they do add a lot of value when it comes to fighting financial crimes like money laundering. This is an area in which the use of machine learning—a process in which machines learn to discover their own algorithms without direct instruction from a human-programmed algorithm—really makes a difference. Here, we examine the use of AI in anti-money laundering (AML) transaction monitoring, as applied in Discai’s KYT AML solution, in line with the six conditions above:
- It solves a real business problem. Due to the ever-increasing complexity of money laundering schemes, as well as increased regulatory scrutiny, FIs are struggling to keep up with anti-money laundering (AML) investigations. First and second line investigations teams are rapidly growing to both maintain traditional rule-based solutions and investigate all resulting alerts.
- The business problem is large and costly. Financial institutions must invest in one way or another, either by expanding their AML investigation teams or investing in sustainable software.
- The problem occurs frequently. Unfortunately, money laundering and other financial crimes happen every day. The United Nations Office on Drugs and Crime (UNODC) estimates that between 2 and 5% of global GDP—up to 1.87 trillion euros—is laundered each year. At the same time, according to a recent study conducted by Europol, only around 2% of the laundered money is actually seized by the authorities. Fortunately, not all of those money launderers are likely to be customers of your bank, but the figures suggest it’s safe to assume these crimes are happening frequently.
- There’s a need for speed. Even in ex-post investigations, a fast investigation might result in an opportunity to limit the damage caused. Moreover, less time spent on false positives means more time to potentially spend on cases with real AML risk.
-
Past money-laundering cases and customer behaviour help to reveal current crimes. As money laundering is becoming more and more complex, FIs cannot rely solely on historical case data to reveal modern money laundering. When evaluating transaction monitoring solutions, they should look at combining several AI techniques in relation to individual customers:
- Unsupervised models detect anomalous behaviour compared to other customers or historical behaviour of the same customer. This indicates that the behaviour of the customer differs from what is expected and deserves the attention of the investigator, and helps to identify types of money laundering that haven’t been seen before.
- Supervised models are trained to detect suspicious behaviour using historical observations of suspicious activity reports. Usually, these patterns are far too complex to be documented in a rule-based transaction monitoring system without creating large numbers of false positives.
- Graph learning can be used to connect the dots between customers in a network of transactions. If a customer regularly transfers money to a known money launderer, it might deserve your attention.
- A human is in the loop. An AI-based AML solution can reduce false positives in AML transaction monitoring, but there will still be some customers that are investigated but not reported to a financial intelligence unit (FIU). In other words, even though the model will provide a rich context and offer ‘explainability’ (that will facilitate the work of an investigator and is required by regulators), its might sometimes be wrong. That’s why we strongly recommend keeping humans involved in the process. These people monitor the model performance, do the actual investigation (augmented by the models), and safeguard the quality of the solution.
How does AI compare to other financial crime-fighting techniques?
Traditionally, banks try to capture money laundering by writing complex scenarios in rule-based transaction-monitoring systems. These scenarios are built using expert input and are able to describe typical behaviour that might be related to money laundering. However, these scenarios are also relatively easy for money launderers to bypass, for example, by cutting transactions in smaller pieces (smurfing), using insurance and securities products, or transferring money between countries, currencies, and jurisdictions. As money launderers are becoming more professional, so are their methods. Trying to keep up with traditional rule-based transaction monitoring systems has a high cost and will be a lost fight in the end. If we want to win, we need to fight with equal arms.
AI might not be the answer to every issue faced by FIs, but it is an excellent tool in the fight against financial crimes like money laundering. If your situation aligns with the conditions described above, you’re likely to benefit from an AI solution. To find out more about how Discai can improve the performance of your AML investigations team, get in touch with us today.
Looking for an AI solution to improve AML investigations?
Get in touch now!