Finding your flavour: alternative methods for identifying potential money laundering
For financial institutions, identifying potential money launderers in a portfolio of customers can be like searching for a needle in a haystack.
In the old days, customers would visit a branch office and meet with a local representative. This rep knew how they typically transacted and could recognise when something suspicious was going on.
Today, customers use digital channels to quickly send money across the globe, while straight-through processing and biometric authentication allow people to become customers in mere minutes. All this digital activity makes it difficult to ‘know your customer’ (KYC) and ‘know your transaction’ (KYT) in the traditional way, but it also generates a lot of data. Finding potential money laundering in the midst of so much information can be a difficult, time-consuming, and frustrating task.
To track down money launderers in the modern age, we need modern solutions.
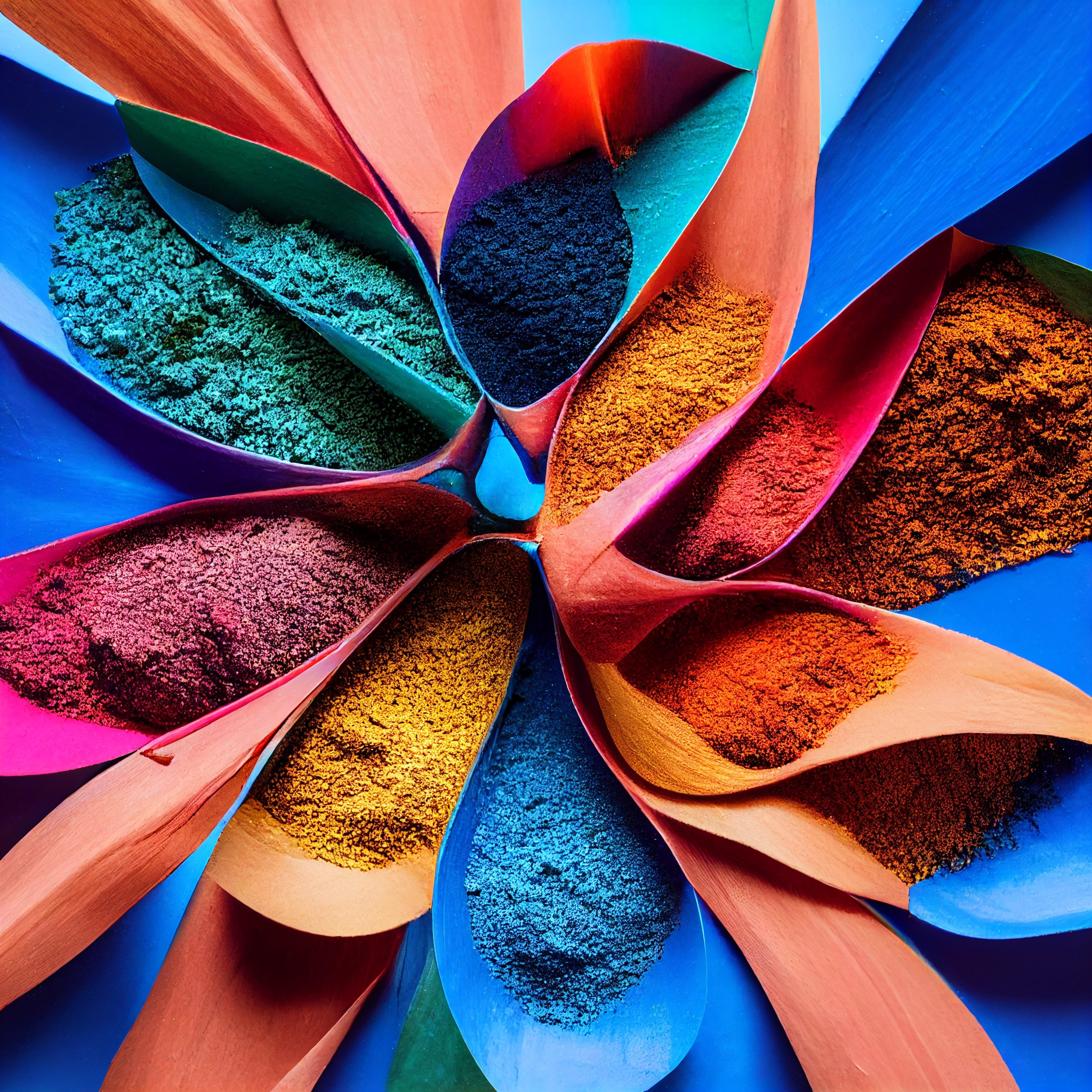
For financial institutions, identifying potential money launderers in a portfolio of customers can be like searching for a needle in a haystack.
In the old days, customers would visit a branch office and meet with a local representative. This rep knew how they typically transacted and could recognise when something suspicious was going on.
Today, customers use digital channels to quickly send money across the globe, while straight-through processing and biometric authentication allow people to become customers in mere minutes. All this digital activity makes it difficult to ‘know your customer’ (KYC) and ‘know your transaction’ (KYT) in the traditional way, but it also generates a lot of data. Finding potential money laundering in the midst of so much information can be a difficult, time-consuming, and frustrating task.
To track down money launderers in the modern age, we need modern solutions.
Note that in this blog:
- We refer to ‘potential money launderers’ as AML (anti-money laundering) cases that are reported by a financial institution to the regulator. These are typically pending further investigation and are not yet proven cases of money laundering.
- We refer to ‘customers’ to be investigated, while in reality, triggers might be active on a customer, transaction or account level.
The two extremes of AML transaction monitoring: rule-based vs AI-based triggers
Rule-based AML triggers
Traditionally, financial institutions have exposed AML cases by creating rules (or ‘scenarios’) that identify suspicious behaviour. These might be relatively simple, for example, a rule that determines all cash withdrawals above €1,000 should be investigated. But they can also be quite complex, using combinations of input variables, or tactics like whitelisting (creating lists of persons or entities that should not be triggered) or other exclusion criteria. This approach has been common practice amongst financial institutions and regulators for years.
The main benefit of using rule-based triggers for AML investigations is that it’s relatively easy to explain why a certain customer has been singled out for investigation. As soon as new types of money laundering are identified—in the news or in the portfolio—a new rule can be implemented to monitor these risks.
But what about the downsides? These rules also trigger investigations into customers that are not laundering money at all (aka ‘false positives’). In general, less than 5% of investigations lead to a customer being reported to the regulator. But of course, the remaining 95% still need to be investigated, demanding time and effort from the AML team. On top of that, as money launderers becoming more and more professional and regulators are raising the bar in terms of AML investigation requirements, the number of rules needed to cover all potential money laundering cases is constantly increasing.
The pros and cons of using rule-based triggers for AML investigation:
AI-based AML triggers
In recent years, the use of artificial intelligence (AI) in the detection of potential money laundering has skyrocketed. As AI is a broad field of science, it takes many forms in the context of AML, such as:
- Anomaly detection – Detecting behaviour that is atypical for the customer in question.
- Network analysis – Detecting interconnections between customers in a network of transactions.
- Risk scoring – Matching similarities in data patterns with historical cases of money laundering (aka ‘supervised learning’).
AI methods are brimming with benefits. Most importantly, they allow financial institutions to reduce the number of investigations required to find the same number of potential money launderers. This means less time spent on investigating false positives and more time spent on actually getting to the bottom of suspicious activity. Plus, when supervised learning is applied, AI models learn from past investigations. This allows them to improve triggers that were previously incorrect or recognise new types of money laundering that have been identified by police investigations, attentive colleagues or anomaly models.
Of course, as a new way of working in a highly regulated context, the use of AI requires a mindset change. Explaining why an investigation into a customer was triggered (or not) is not as straightforward as in the traditional approach, but thankfully, methods are available to add ‘explainability’ to AI models. These are able to identify which variables have been important for the model to activate the trigger. In practice, the explainability output could include variables like ‘the number of cash withdrawals above €10,000 in the last six months’ or ‘the amount sent to high-risk AML countries in the last month’.
The pros and cons of using AI-based triggers for AML investigation:
Finding the optimal approach: baby steps or a great leap forward?
In reality, there are many shades of grey between these two extremes. It’s important for every financial institution to find out what works best for their organisation and regulatory context, accounting for capacity, data maturity and risk appetite.
In most countries, there is a long tradition of using rule-based triggers, making it a challenge to switch to AI-based solutions. In progressive countries, using AI to find potential money launderers is starting to become commonplace, so it’s not such a leap. But in other, more conservative countries, it might be wise to take an intermediate step.
A spectrum of hybrid solutions
Some AML risk engines offer a combination of using AI for efficient and effective handling of alerts and rule-based alerts for certainty on the coverage of AML risk types. In these hybrid solutions, several ‘flavours’ are possible.
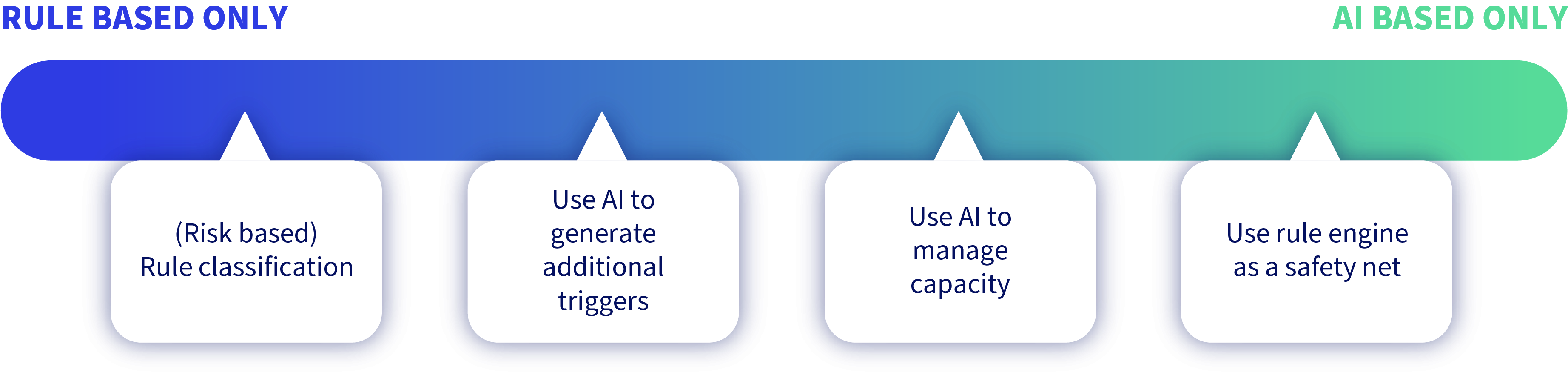
Risk-based rule classification
In risk-based rule classification, rules are created in the traditional way: based on customer, transaction and/or account characteristics. All of these resulting triggers are investigated by the AML team. However, AI models are used to ensure that the triggers with the highest likelihood of potential money laundering are attended to first. This means if the AML team is not able to investigate every trigger in a timely manner, the backlogs will contain a minimum of true potential money launderers. But there’s also a downside. Potential money launderers might be identified by the AI (via a high risk score), but not investigated because no rule has been triggered.
AI for the generation of additional triggers
In this approach, all rule-based triggers are offered for investigation and prioritised based on their (AI-based) risk score. However, on top of the rule-based triggers, extra triggers are created for customers that score above a certain risk threshold. This can be driven by anomaly models, network relations, supervised machine-learning models, or other types of AI.
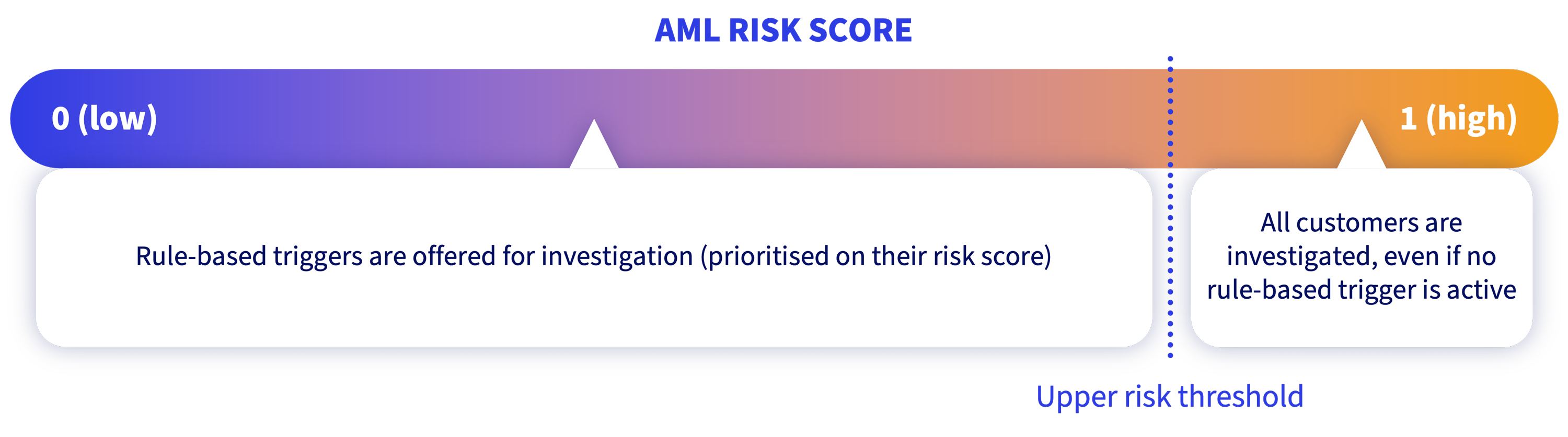
This approach empowers the AML team to identify many potential money launderers. The number of customers to be investigated tends to grow rapidly, but by combining this approach with risk-based rule classification, the most pertinent cases are investigated first. So, if there are backlogs, they’ll mostly contain false positives.
AI for managing capacity
If we go one step further along the spectrum, AI model scores can be used to determine which rule-based AML triggers need to be investigated. Typically, two risk thresholds are set, based on the capacity of the AML team.
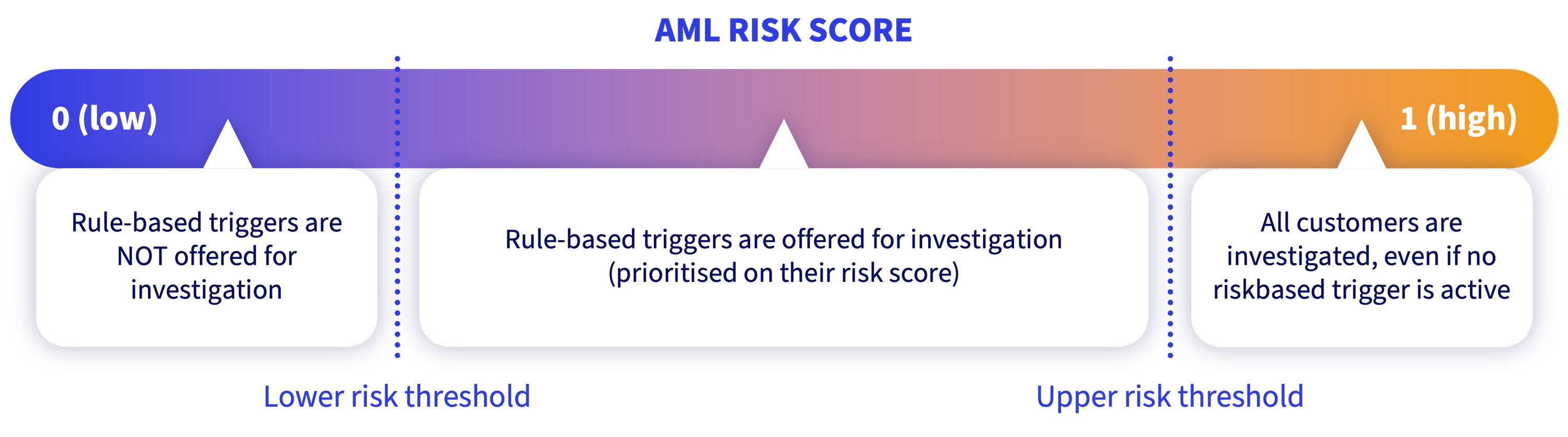
All customers that score above the upper threshold are still being investigated, capitalising on the ability of AI models to find potential money launderers based on complex data patterns. Of all the rule-based triggers that are activated, only the customers that score above the minimum threshold will be investigated. In this way, long backlogs are avoided, and the AML team spends less time investigating false positives.
Note that several alternative hybrid solutions are possible. One of the most common alternatives available in the market is to use AI to automatically close or hibernate triggers that were created by a traditional (rule-based) system. Although this approach certainly has its benefits (mainly a smaller change for both the investigator and the regulator, as well as a first improvement in efficiency), it is generally considered suboptimal. The main reasons for this are that it
- does not address the false negative problem,
- does not identify new types of money laundering (new rules are to be created manually),
- and requires significant (rule) maintenance and (model) retraining effort.
Rule engines as safety nets
In more progressive markets and organisations, AI models can be used to determine which accounts, customers or transactions are triggered for investigation. This approach offers the highest level of efficiency in the investigation process and, at the same time, ensures that lessons learned from past investigations throughout the organization are automatically incorporated. This includes alerts that were created by police investigations or attentive colleagues in the commercial (branch) network.
In this approach, coverage assessments are usually performed to assess whether all AML risk typologies are covered by the AI solution. Rule engines can be used as a safety net, accounting for risk types that are not sufficiently covered by the models. Typical reasons for this are that the AML risk does not occur frequently enough (and therefore might not be covered by a supervised machine learning model) or that a particular investigation is required by the regulator.
So, where does Discai fit in?
Discai’s KYT AML solution is built in such a way that it can be integrated across the spectrum of previously discussed hybrid solutions. Although Discai offers a rule engine as a safety net, we also unleash the potential of AI models for our customers. We partner with you to find the best approach for your organisation, in line with its risk appetite, data maturity, team capacity, and regulatory context.
Ready to boost the performance of your AML investigations, using AI?
Discover the benefits of artificial intelligence